- Last modified: July 3, 2024
Dennis Nenno, a former quantum physicist turned software executive, offered a nuanced perspective recently on the intersection of quantum computing and machine learning (ML). While acknowledging the current limitations of quantum technology, Nenno suggested that its potential applications in ML could materialize sooner than many expect.
Nenno began by addressing the current state of quantum computing: “Even today nobody has come up with an actually useful application I guess except Google a couple years ago. They published a paper which they coined the term quantum supremacy.”
This reference to Google’s 2019 quantum supremacy claim underscores the nascent nature of the field, where practical applications remain elusive.
However, Nenno sees promise in quantum computing’s ability to accelerate certain ML algorithms.
“For machine learning it’s been shown now that you can speed up exponentially a number of very important algorithms,” he said. One such algorithm is principal component analysis, which is crucial for recommendation systems like those used by Netflix.
The potential of quantum memory, or “Quantum RAM,” is particularly intriguing. Nenno explained: “Thirty quantum bits (qubits) can store the equivalent of gigabits of classical data, while 40 qubits can already store the equivalent of terabits of classical data.” This exponential storage capacity could revolutionize the scale of machine learning problems that can be tackled.
Despite these promising developments, Nenno acknowledged significant challenges, particularly in data transfer between classical and quantum systems.
“The next challenge is well how do you get that classical data onto a quantum computer that’s a super hard problem to figure out and how to get it back is another problem to figure out,” he said.
Interestingly, Nenno suggested that the inherent noise in quantum systems might actually be beneficial for certain machine learning techniques.
“We have limited control over these quantum systems due to noise,” he began. “However, this noise can be beneficial in applications like stochastic gradient descent, which is one of the main drivers of neural networks.”
In his closing remarks, Nenno offered a provocative prediction: “I believe that we get quantum machine learning earlier than anybody will be able to crack quantum encryption which is where most of the money goes now.”
This suggests that practical applications of quantum computing in ML may emerge sooner than anticipated, potentially outpacing progress in quantum cryptography.
While quantum computing’s impact on ML remains speculative, Nenno’s ideas suggest that this intersection could be a fertile ground for innovation, potentially delivering practical benefits before other highly anticipated quantum applications.
Featured image: Credit: Ignite
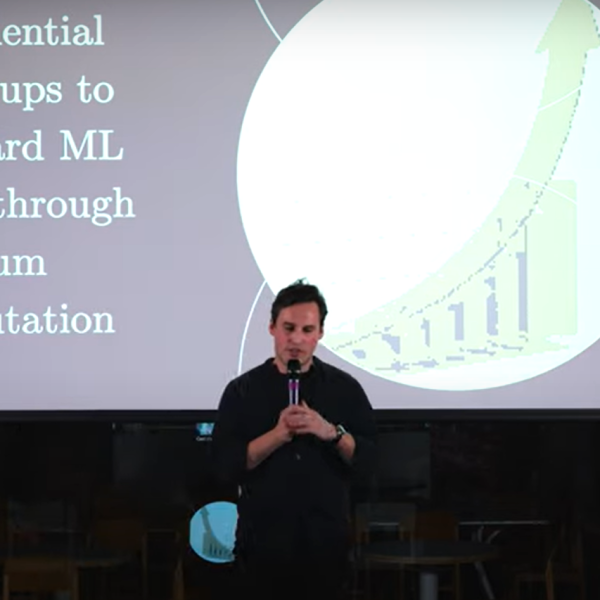