Insider Brief
- AI Insider has introduced a seven-layer market mapping framework to help organizations address fragmentation and misalignment in the rapidly expanding AI ecosystem.
- The framework maps over 450 technologies by how AI systems are actually built and deployed, covering layers from infrastructure to governance.
- Without a system-wide view, companies risk making siloed decisions—such as investing in model training without scalable inference—leading to stalled deployments and wasted resources.
As artificial intelligence adoption accelerates, many companies are learning a hard lesson: technical ambition alone doesn’t guarantee results. From stalled deployments to costly vendor misfires, one of the biggest risks in AI development today is a lack of system-wide understanding, according to a new report from The AI Insider.
Common failure points are now well-known. Models are trained but can’t be deployed at scale. Orchestration tools are installed without the data infrastructure to support them. Security is addressed only after systems go live. These misalignments often stem from a single source—fragmented decision-making.
Siloed Thinking Can Be Structural
The problem may be structural, the analysts suggest. Most AI market maps focus on narrow segments of the ecosystem, such as developer tools, models, or applications. As a result, organizations and investors frequently make decisions in silos, missing critical dependencies between layers. Investments go to trending technologies rather than those required to support long-term, scalable systems.
AI Insider, a research and intelligence firm, is taking a different approach. Its newly released seven-layer market mapping framework aims to reorient how organizations see the AI stack—not as a loose collection of tools, but as an integrated, layered system. The model organizes more than 450 AI technologies according to how they are actually built, deployed, and maintained in production environments.
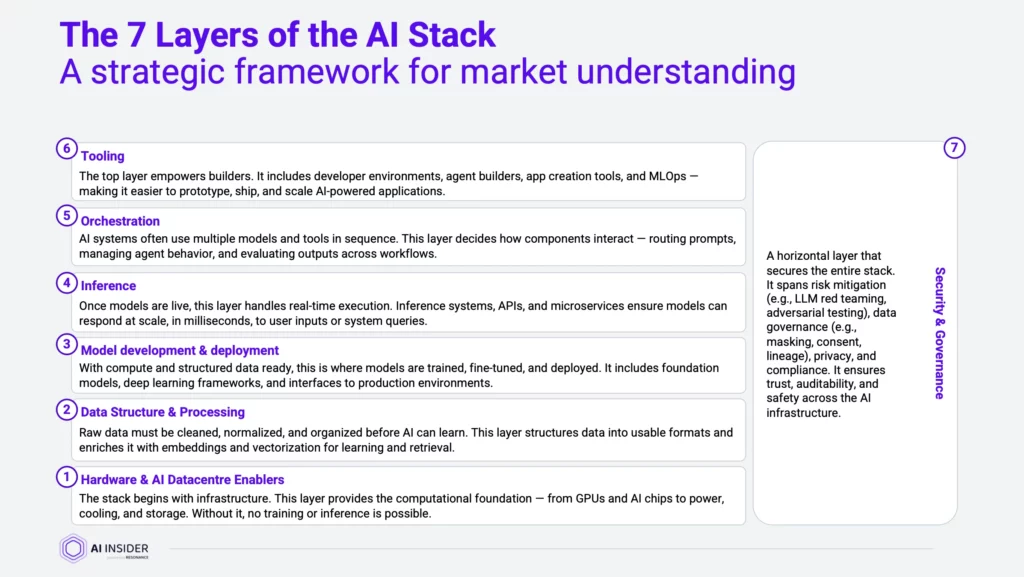
New AI Framework
The framework begins at the physical layer—hardware and datacenter infrastructure—and moves upward through data processing, model development, inference, orchestration, tooling, and finally governance. Each layer plays a distinct role, and breakdowns at one level can derail progress across the stack.
The first layer, hardware and compute infrastructure, includes the physical systems — such as GPUs, networking, and cooling — that support AI workloads. Providers like NVIDIA, AMD, AWS, and Oracle dominate this space. Without stable power systems or high-speed connectivity, higher-order AI processes simply cannot function reliably.
Next is data structure and processing, where raw data is transformed into usable formats for machine learning. This step, handled by vendors such as Pinecone and Tecton, directly impacts the accuracy and reliability of downstream models. Poor data quality at this stage will ripple through the entire stack.
The third layer focuses on model development and deployment, where training and fine-tuning occur. Players such as OpenAI, Hugging Face, PyTorch, and TensorFlow operate here. However, even the most advanced models need efficient deployment pipelines to be usable in live applications.
Inference, the fourth layer, enables real-time application of models in production. It’s where AI systems interact with users—delivering outputs via APIs or microservices architectures. Delays or instability here affect user experience and operational efficiency.
Beyond inference, orchestration tools — such as LangChain, MLflow, and Run.ai — allow organizations to chain models together, route prompts and manage decision-making pipelines. But these capabilities rely on a stable foundation underneath. Deployed too early, they offer little value.
The tooling layer enables development and monitoring, while the final layer — security and governance — spans all others. Here, companies like Skyflow, DataRobot, and Trustible focus on traceability, compliance, and adversarial testing, increasingly critical in regulated industries.
More Than a Vendor List
By mapping the ecosystem this way, AI Insider offers organizations more than just a list of vendors—it provides a structured view of how AI systems actually work in practice. Analysts argue this helps enterprises avoid overinvesting in flashy but disconnected components, and instead sequence their adoption strategies to match real-world technology flows.
It also allows businesses to assess market saturation, spot underinvested areas, and align teams across functions. A startup may decide to avoid the crowded chatbot layer in favor of secure agent infrastructure. A healthcare organization might prioritize data lineage and model traceability from day one to meet compliance needs.
The firm plans to release deep-dive analyses of each layer in future reports. For now, it says the layered model provides a strategic lens to correct the fragmented thinking that often derails AI initiatives. For companies building AI systems in 2025 and beyond, seeing the full stack may be the clearest path to long-term success.
Click here to download full version of the report.