- Last modified: June 13, 2024
Insider Brief
- Drugs and vaccines discovered through artificial intelligence achieved an 80-90% success rate in Phase I clinical trials.
- Based on limited data, the success dropped rate to around 40% in the Phase II, but seems in line with industry standards.
- The findings were published in the peer-reviewed Drug Discovery Today.
A team of researchers from the Boston Consulting Group report that drugs and vaccines discovered through artificial intelligence achieved an 80-90% success rate in Phase I clinical trials – far exceeding historic industry averages of 60% – though success dropped to around 40% in the limited Phase II data. Though lower, the Phase II rate seems — at least so far — in line with industry standards for those trials, the analysts added.
“In Phase I trials, AI-derived molecules can have a success rate of 80–90%, which is substantially higher success rates than historic averages,” the team states. “This suggests that AI is highly capable of designing or identifying molecules with drug-like properties.”
The new findings, published in the peer-reviewed Drug Discovery Today, provide a comprehensive look at the clinical pipelines of biotechnology companies specializing in AI-powered drug discovery. By aggregating data across dozens of drug candidates, the researchers found compelling evidence that AI techniques may be capable of routinely identifying molecules with promising drug-like properties.
“Our findings show that benefits are beginning to manifest in clinical trials as well,” the researchers state. “Despite the statistical caveats, we believe these results paint an intriguing picture for the future of R&D.”
In Phase I clinical trials, which test a drug’s safety profile in healthy volunteers, the analysis found that 80-90% of AI-discovered molecules successfully passed this stage and progressed to later trials. This is a stark contrast to the historic industry average Phase I success rate of only 60%.
The superior performance of AI-derived candidates persisted even for molecules targeting novel biological pathways, indicating these results cannot be simply explained by AI companies pursuing well-trodden biological targets.
While the success rates for AI-derived molecules dropped in later-stage trials, the researchers note this data is still quite limited. In Phase II, where a drug’s efficacy is first evaluated, around 40% of AI-discovered molecules were successful – comparable to industry averages.
“In the coming years, as more clinical results for AI-discovered molecules become available, it will be exciting to see how AI techniques will impact R&D productivity overall,” the team writes.
The findings arrive at a pivotal moment when most major pharmaceutical companies have launched AI-focused drug discovery initiatives, often in partnership with AI-native biotechnology firms. Deal-making in this space has accelerated rapidly over the past five years as the industry races to leverage AI/machine learning capabilities.
For years, the widespread adoption of AI in pharmaceuticals has been clouded by uncertainty around whether these computational approaches could consistently deliver actionable results. While individual successes have occurred, a comprehensive assessment of AI’s clinical impact has been lacking until now.
“This improvement in success rates could be due to several different reasons,” the researchers note. “One reason could be that AI discovery efforts pursue well-validated biological targets and pathways, which reduces the risk of on-target toxicity. Although this might play a part, we are seeing early signs of molecules going after novel targets passing through Phase I.”
They add that, based on this, the high success rate in Phase I is not just the result of going after well-validated biology.
As mentioned, the researchers say there are limitations to their study. The sample sizes, especially for later-stage clinical trials, are still quite small, so the success rates could change substantially as more data emerges over the coming years. Additionally, the study only included molecules from AI-native biotechs and partnerships, excluding those originating purely from large pharma companies’ internal AI efforts. There are also some nuances around categorizing molecules that used multiple AI techniques. The researchers call out these caveats in the discussion of how they may impact the conclusions drawn.
The researchers emphasize that much larger datasets over longer timeframes will be required to fully assess AI’s impact on clinical attrition and overall R&D productivity.
The team members include: Madura KP Jayatunga, Margaret Ayers, Lotte Bruens, Dhruv Jayanth and Christoph Meier.
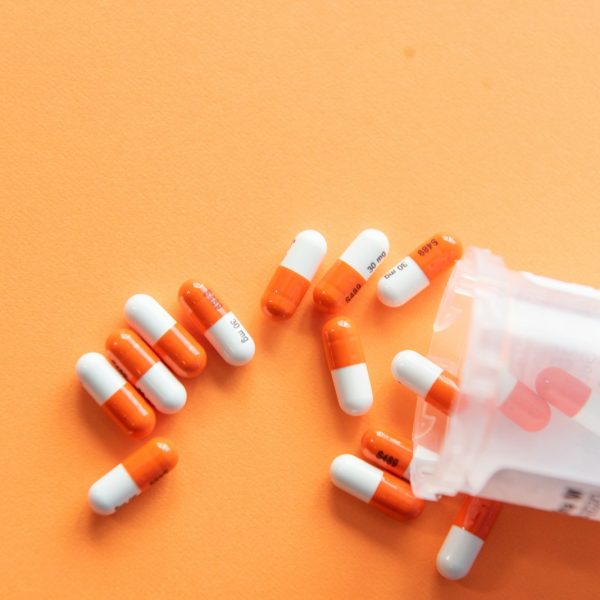