- Last modified: June 26, 2024
In a recent interview with Lex Fridman, AI researcher, co-founder and CEO of Perplexity Aravind Srinivas provided a concise yet comprehensive overview of the major breakthroughs that have shaped the current landscape of artificial intelligence (AI), particularly in the realm of language models.
Srinivas traced the evolution from early neural network architectures to the game-changing Transformer model, highlighting key innovations along the way. He explained how attention mechanisms, initially introduced by Yoshua Bengio and colleagues, proved to be a crucial step forward. The real breakthrough, however, came with the development of self-attention and its implementation in the Transformer architecture.
“Google Brain’s research, along with Wasani ATL, identified that it’s okay to take the good elements of both; it’s more powerful than convolutions. It learns more higher-order dependencies because it applies more multiplicative compute,” said Srinivas noted, stressing the Transformer’s ability to efficiently process information in parallel.
This architectural innovation paved the way for increasingly large and capable language models. Srinivas outlined the progression from GPT-1 to GPT-3, describing how each iteration leveraged more data and computing power to achieve better performance. He pointed out that the focus shifted more towards components outside the architecture, such as the data used for training, the nature of the tokens, and their density.
While discussing recent advancements, Srinivas touched on the importance of post-training techniques like Reinforcement Learning from Human Feedback (RLHF). He explained: “without good post-training, you’re not going to have a good product but at the same time without good pre-training there’s not enough common sense to like actually have you know have the post training have any effect.”
Looking to the future, Srinivas explored the potential for more efficient AI systems that can reason effectively without relying on massive pre-training. He described ongoing research into smaller language models (SLMs) that focus specifically on reasoning capabilities: “if we do manage to somehow get to a right data set mix that gives good reasoning skills for a small model then that’s like a breakthrough that disrupts the whole Foundation model players.”
The conversation between Srinivas and Fridman offers valuable insights into the rapid progress of AI technology, from the fundamental breakthroughs in neural network architectures to the current focus on enhancing reasoning abilities and efficiency. As the field continues to evolve, these developments promise to shape the future of artificial intelligence in profound ways.
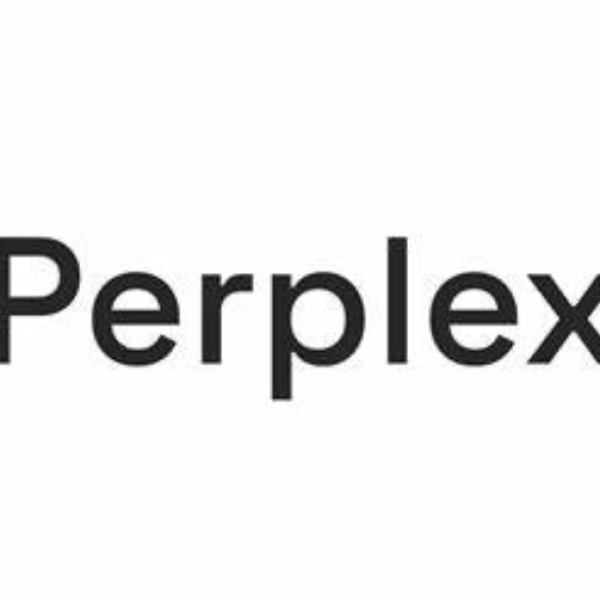