Insider Brief
- AI Insider’s Market Map: Hardware & Datacenter Enablers breaks down the hardware and infrastructure layer of the AI stack, revealing how datacenters, chips, power systems, and networking gear form the physical foundation for scaling artificial intelligence.
- The analysis highlights nine key categories—ranging from GPUs and AI chips to power and cooling—emphasizing how performance, sustainability, and scalability challenges shape this industrial backbone.
- While dominated by global incumbents, the space is seeing early disruption from startups in modular energy and edge AI chips, signaling new opportunities in a capital-intensive and strategically critical layer of the AI ecosystem.
- You can download the Hardware & Datacenter Enablers market map here.
AI Insider’s seven-layer AI Stack market map was designed to bring structure to a fragmented ecosystem. In our first post, we laid out the full-stack framework – spanning hardware, data infrastructure, model development, orchestration, and governance – to help organizations and investors understand how AI systems are actually built, scaled and secured. The idea was simple: you can’t make smart decisions if you only see part of the stack.
In this follow-up, we’ll begin unpacking the stack layer by layer, starting with the physical foundations: AI datacenters, chips, and the infrastructure that powers real-world workloads. Each post in this series will focus on a single layer, highlight standout companies, and explore where innovation is heating up — and where risks are emerging. Whether you’re navigating cloud procurement, edge inference, or sustainability mandates, our goal is to equip you with strategic insight from the bottom up.
Securing Scalability, Coordinating Complexity
As AI models grow in complexity, so too does the machinery required to run them. Beneath the surface of every chatbot, recommendation engine, or self-driving system lies a vast and intricate physical infrastructure, an ecosystem of energy systems, cooling networks, high-performance processors, and storage clusters, all humming in coordination.
To make sense of this technical sprawl, AI Insider’s Market Map: Hardware & AI Datacenter Enablers lays out the architecture powering artificial intelligence from the ground up. It’s a snapshot of the companies responsible not for training large language models or building flashy apps, but for building and maintaining the industrial scaffolding that makes those tools possible.
Split across nine categories, the market map breaks down the layered stack supporting AI workloads—starting with energy and ending with virtualization. It includes both industry incumbents and rising players, spanning power engineering firms, chip startups, legacy data infrastructure vendors, and edge computing innovators.
Together, these firms form the unsung backbone of the AI revolution.
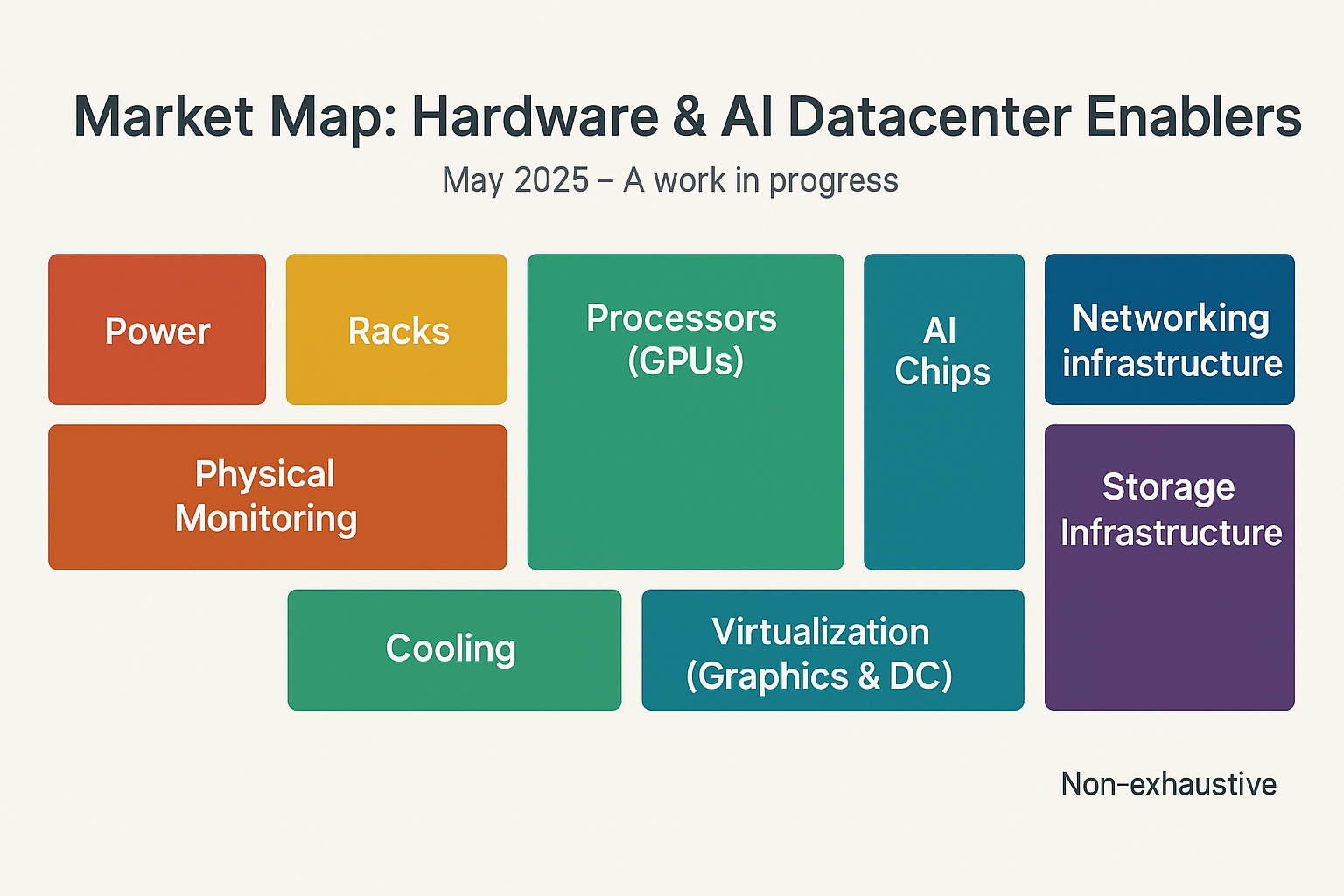
- Power: The First Link in the Chain
All computation begins with power. AI workloads – such as training large language models and autonomous driving platforms – are energy-intensive, and ensuring a continuous power supply is mission-critical. Datacenters now require industrial-grade solutions – redundant power distribution units, battery backups, and increasingly, new forms of energy generation.
Established players like Schneider Electric, Eaton, and ABB offer power management systems, while newer entrants like NuScale and Kairos Power explore modular nuclear reactors as future-proof solutions. As demand grows, energy isn’t just a utility—it’s becoming a competitive differentiator.
- Racks: Physical Structure for Compute Clusters
Next comes the physical housing. Racks hold the servers, chips, and storage systems in modular units optimized for airflow, density, and cable management.
Companies like Supermicro, Vertiv, and Lenovo build and sell the structural core of datacenters. These racks aren’t passive furniture—they’re critical for managing heat, reducing cable complexity, and scaling efficiently as AI deployments expand from dozens to thousands of compute units.
- GPUs: The Muscle Behind Machine Learning
GPUs (Graphics Processing Units) are the backbone of modern AI computation. Originally designed to render graphics through parallel processing, they are now central to AI because they excel at handling the massive matrix operations required by machine learning—especially deep learning.
In practice, GPUs are used in two critical phases of the AI lifecycle:
- Training, when models are “taught” by processing enormous datasets, requiring high computational throughput.
- Inference, when trained models are deployed to make real-time predictions—whether that’s generating text, identifying images, or making recommendations.
NVIDIA, AMD, and Intel dominate this space, offering GPUs tuned for training and inference. Companies like Apple and Samsung also appear on the map, reflecting their growing focus on AI-optimized chips for mobile and edge devices. These processors drive everything from recommendation algorithms to autonomous vehicles.
- AI Chips: Customized Intelligence Engines
AI chips (also known as AI accelerators or custom silicon) are processors specifically designed for AI tasks—engineered to execute neural network operations faster, more efficiently, and with greater power economy than general-purpose GPUs or CPUs.
While AI chips are unlikely to replace GPUs across the board in the near term, they are increasingly viewed as complementary—particularly in edge environments or scenarios with specific performance, latency, or power constraints
Startups like Cerebras and Graphcore are designing ultra-specialized silicon optimized for massive AI models. Meanwhile, hyperscalers like Google, Amazon, and Microsoft are producing proprietary chips like TPUs and Trainium to reduce reliance on third-party suppliers and better align hardware with their software stacks.
Others, like Tenstorrent and FuriosaAI, are building edge-focused chips, aiming to deliver fast inference with minimal power draw—ideal for devices like drones, cars, or mobile phones.
- Cooling: Battling the Heat
High-performance chips generate enormous amounts of heat. Without proper cooling, performance drops and hardware fails. The cooling ecosystem includes traditional air systems as well as more advanced liquid and immersion cooling technologies.
Iceotope and LiquidStack lead in liquid cooling innovation, providing solutions for high-density clusters. Schneider Electric and Vertiv, already active in power and racks, also offer integrated thermal management systems. As chip density rises and environmental pressure mounts, cooling efficiency will become both a technical and sustainability issue.
- Networking Infrastructure: Moving Data at Scale
Computation doesn’t happen in isolation. Networking infrastructure connects nodes, moves training data, and enables real-time inference across distributed environments. It’s the circulatory system of the AI stack.
Companies like Cisco, Arista, and Juniper provide high-throughput, low-latency networking gear, often relying on specialized protocols like InfiniBand or 400G Ethernet. Broadcom and Mellanox Technologies (acquired by NVIDIA) also play key roles in hardware and switching technology to enable rapid, reliable communication across GPUs and storage nodes.
- Storage Infrastructure: Holding the Data That Trains AI
AI needs vast amounts of data—and it needs to be fast, secure, and constantly accessible. Storage infrastructure ensures that datasets, model weights, logs, and inference outputs are safely and efficiently housed.
Firms like Western Digital, Pure Storage, and NetApp provide the backbone for this layer, offering HDDs, SSDs, and flash arrays tuned for durability and high-speed access. Hewlett Packard Enterprise, IBM, and Dell round out the category with hybrid solutions that blend performance and capacity.
Storage is no longer just a back-office concern—it’s essential for performance tuning and cost optimization.
- Physical Monitoring: Watching the System
To keep these datacenters running, operators need real-time telemetry—tools that track temperature, power consumption, rack status, and early signs of hardware failure.
Companies like Honeywell, LogicMonitor, and Zabbix offer monitoring platforms that integrate into broader datacenter management systems. These platforms collect and analyze data across thousands of endpoints, providing early alerts and automating fault response.
Vaisala and RF Code add environmental monitoring, while APC and Room Alert provide rack-specific sensors for localized diagnostics.
Monitoring isn’t just about preventing outages—it’s about optimizing uptime and operational efficiency.
- Virtualization: Making Compute Flexible
The final layer is virtualization, which allows hardware to be abstracted into shareable compute units. Through containers, virtual GPUs (vGPUs), or orchestrated environments like Kubernetes, workloads can be spun up, assigned, or shifted across hardware without downtime.
VMware, Red Hat, and Oracle are long-time leaders in enterprise virtualization. Intel, NVIDIA, and Citrix offer GPU-specific solutions. Startups like Virtuozzo and tools like Scale Computing bring flexibility to smaller and edge deployments.
Virtualization enables AI to run efficiently across different environments—cloud, on-premise, or hybrid—while maximizing hardware utilization and reducing cost.
Hardware Is the Next Competitive Frontier
AI may be powered by algorithms, but those algorithms are only as good as the infrastructure that supports them. This map reveals a strategic shift: from thinking of AI as a software-only industry to understanding it as an industrial system, built and optimized like a power grid or logistics network.
As model complexity rises and deployment scales globally, companies that master this stack—from power to virtualization—will gain more than technical advantage. They’ll own the rails on which future AI applications will run.
In our next article, we will review the second layer of AI Insider’s Seven Layer Full-Stack AI Map: Data Structure and Processing. Before AI can learn, the data has to make sense. This layer of the stack handles the transformation of raw, messy inputs into structured formats machines can understand. Companies in this category focus on cleaning, labeling, normalizing, and embedding data — turning it into something usable for model training or retrieval. Without this step, even the most advanced models risk learning from noise.
Strategic Observations from the Market Map
The Hardware & AI Datacenter Enablers layer varies from the rest of the AI stack—both in structure and in business dynamics. Unlike software-centric layers where startup activity is accelerating, this foundational layer is shaped by industrial scale, deep capital intensity, and long technology cycles. The market remains largely concentrated in the hands of established multinationals—especially in the U.S. and Asia—spanning power systems, chip manufacturing, racks, cooling, and networking. These are companies with significant engineering moats and global supply chains, and their dominance reflects the sheer complexity and capital demands of operating at this level.
That said, important exceptions are beginning to emerge. In the power category, we’re seeing early-stage players—particularly in modular nuclear and alternative energy—begin to challenge the status quo. Similarly, niche startups in edge AI chips are introducing highly specialized silicon, targeting low-power inference at the edge where agility and efficiency matter more than scale.
These patterns suggest that while the layer is less fragmented than others, it is no less strategic. In fact, it may be the most consequential: any bottleneck in power, thermal management, or compute availability creates ripple effects across the entire stack. As model sizes grow and sustainability pressures mount, organizations that actively understand and engage with this physical layer will be better positioned to reduce operational risk, unlock scalability, and compete on performance and reliability.
You can click here for the Full Market Map: Hardware And AI Datacenter Enablers.
For more information, or to connect with our analysts, contact us directly at [email protected]
This the market map for Layer 1 of our 7-layer AI stack framework. We will be discussing remaining six layers will be revealed in the coming weeks, each one zooming in on a critical part of the AI value chain
Appendix: Sample Companies Across Layer 1
- Power
- Racks
- GPUs
- AI Chips
- Cooling
- Network infrastructure
- Storage infrastructure
- Physical monitoring
- Virtualization